Material phase classification by mears of Support Vector Machines.
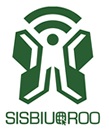
Date
2018Author
Ortegon Aguilar, Jaime Silverio
Ledesma Alonso, Rene
Barbosa Pool, Gliserio Romeli
Vazquez Castillo, Javier
Castillo Atoche, Alejandro Arturo
Metadata
Show full item recordAbstract
The pixel's classi_cation of images obtained from random heterogeneous materials is a relevant step to compute their physical properties, like E_ective Transport Coecients (ETC), during a characterization process as stochastic reconstruction. A bad classi_cation will impact on the computed properties; however, the literature on the topic discusses mainly the correlation functions or the properties formulae, giving little or no attention to the classi_cation; authors mention either the use of a threshold or, in few cases, the use of Otsu's method. This paper presents a classi_cation approach based on Support Vector Machines (SVM) and a comparison with the Otsu's-based approach, based on accuracy and precision. The data used for the SVM training are the key for a better classi_cation; these data are the grayscale value, the magnitude and direction of pixels gradient. For instance, in the case study, the accuracy of the pixel's classi_cation is 77.6% for the SVM method and 40.9% for Otsu's method. Finally, a discussion about the impact on the correlation functions is presented in order to show the bene_ts of the proposal.
Collections
- Artículos [196]